From Data to Decisions: How Predictive Analytics is Reshaping Emergency Preparedness in Hospitals
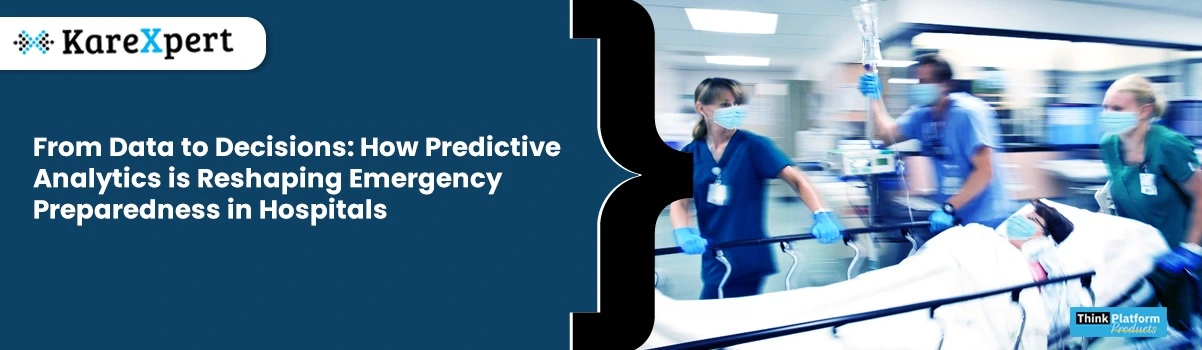
Introduction: Preparing for a New Era—The Age of Emerging Infectious Diseases
With a world growing ever more defined by rapid change and crisis, hospitals are being called to remain prepared for defining emergencies. By disasters, COVID-19 strikes, and mass disasters, they will face their swift response system, which means life or death. The ability to harness predictive analytics, the art and science of analyzing current and historical data to make predictions about future events, represents a game-changing tool in this space. This ability to harness data will allow hospitals to move from a reactive response to a proactive strategy, improving their capacity to protect both patients and staff while conservatively managing high-demand resources.
The Old Struggles of Emergency Preparedness
The traditional approach to emergency preparedness in hospitals is complex, reactive, and faced with many challenges:
Demand Volatility: Hospitals face acute problems in predicting how many patients will come during emergencies, creating overworked staff and limited resources.
Resource Allocation: Reliable data and insight are essential to make effective decisions about the allocation of beds, equipment, and staff.
Communication Gaps: During crises, the coordination between internal departments and outsourcing agencies takes a longer time and is not effectively done.
Limited Scalability: Old models are not flexible enough to quickly scale operations according to the sudden increase in patient volume.
Predictive analytics are breaking through these traditional boundaries by enabling hospitals to foresee and prepare for emergencies like never before.
What is Predictive Analytics?
Predictive analytics is the utilization of data, statistical algorithms, and machine learning techniques to identify the likelihood of future outcomes based on historical data. Predictive analytics was defined as a method of predicting events or trends by extracting information from data and applying machine learning algorithms to these datasets in the hospital setting, where data can be collated from various sources, including electronic medical records (EMRs), historical patient data, staffing schedules, and external data such as weather reports or disease surveillance systems.
Diving Deeper: Predictive Analytics in Emergency Preparedness
Demand Forecasting
– Pandemic Response: Predictive models were employed to determine the number of ICU beds, ventilators, and medical workers needed in specific areas for COVID-19.
– Seasonal Fluctuations: Healthcare facilities know that seasonal spikes in flu-related admissions occur in winter and ante up resources accordingly.
Resource Optimization
– Inventory Management: Maintain sufficient inventories for essential items such as PPE, medications, units of blood, etc.
– Staffing Models: Utilize predictive scheduling to determine peak times for demand and staff accordingly, preventing employee burnout and ensuring adequate coverage.
Disease Outbreak Detection
– Real-Time Surveillance: Hospitals can detect clusters of symptoms that may signal an outbreak by monitoring real-time data on patients.
– Early Alerts: By integrating public health data, hospitals can receive warnings about health crises on the horizon in their zip code.
Disaster Response Planning
– Evacuation Models: Simulations design evacuation models, determining the optimal routes while reducing interruptions.
– Bed Capacity Planning: Predictive models estimate the number of beds required based on the scale and nature of the disaster.
Operational Efficiency
– Emergency Room (ER) Flow: Algorithms predict what time to expect bottlenecks in ERs and make real-time recommendations to improve patient flow.
– Collaboration at Scale: Predictive models improve interdepartmental coordination and help to efficiently transfer critical patients.
Key Case Studies: Predictive Analytics in Practice
U.S. Hurricane Preparedness:
Hospitals in Texas used predictive analytics during Hurricane Harvey to predict surges in patients and allocate resources ahead of time. Data from past hurricanes, weather predictions, and transportation trends enabled hospitals to ready emergency rooms and stockpile workers and supplies.
COVID-19 Oxygen Shortages:
During the second wave of COVID-19, Indian hospitals used predictive models to prepare for oxygen shortages. Predictive analytics was extremely helpful for the optimization of distribution networks and on-time delivery of medical oxygen to hospitals.
Disease Surveillance in Africa:
In Kenya, predictive analytics is used to track malaria outbreaks by analyzing patterns in the climate, patient data, and vector habitats. By identifying these clusters, hospitals have time to prepare for potential spikes in malaria cases and intervene to treat patients in a timely manner.
Advantages of Using Predictive Analytics for Emergency Preparedness
Proactive Decision-Making: Predictive analytics shifts the decision-making paradigm from reactive to proactive, allowing for anticipation of challenges and action taken to mitigate them before they escalate.
Improved Patient Outcomes: Improved resource allocation and emergency management enable early intervention, resulting in better patient outcomes with shorter wait times.
Cost Efficiency: The cost of emergencies on finances is mitigated as predictive analytics minimizes resource wastage by optimizing resource utilization.
Enhanced Staff Morale: Predictive insights enable better distribution of workloads, reducing burnout and preparing staff for potential emergencies.
Strengthened Community Trust: Hospitals that show they are prepared for the worst reassure communities that they can be trusted as providers of comprehensive healthcare.
Challenges and Solutions
Data Silos:
Challenge: Many hospitals suffer from siloed data across multiple systems.
Solution: Invest in unified data platforms and integration tools to create a single source of truth across the organization.
Technological Barriers:
Challenge: Smaller centers, which do not have the infrastructure to support predictive analytics, may not adopt it.
Solution: Cloud-based predictive tools with scalable and affordable options for resource-limited settings.
Data Privacy Concerns:
Challenge: Using patient data presents privacy concerns.
Solution: Follow data protection laws and use encryption along with secure data-sharing methods.
A Glimpse into the Future of Predictive Analytics in Emergency Preparedness
Advanced Algorithms: More accurate predictive models based on advanced algorithms will provide even better forecasts.
Wearable Technology: Patient health will be monitored through data collected using wearable technology, which will help detect emergencies at the earliest.
International Data Sharing: Across nations, hospitals will adopt emergency preparedness principles given evidence for best practices gleaned from global trends.
IoT Integration: The Internet of Things (IoT) will enable hospitals to monitor equipment, patient vitals, and facility conditions in real-time for better operational readiness.
Conclusion: Prediction Should Lead to Preparedness
Predictive analytics is more than a tool — it represents a paradigm change in how hospitals consider their emergency preparedness. Predictive analytics is revolutionizing the healthcare landscape by enabling providers to use data to forecast challenges, optimize resource utilization, and enhance patient care.
In the age of systemic crises, predictive hospitals can help save lives, lower costs, and lay the foundation for a more resilient healthcare system. As this technology improves, we can look forward to an age of preparedness not just in theory but in practice.