DON’T Believe the Hype: Why Not All Predictive Analytics Tools Are Built for Healthcare
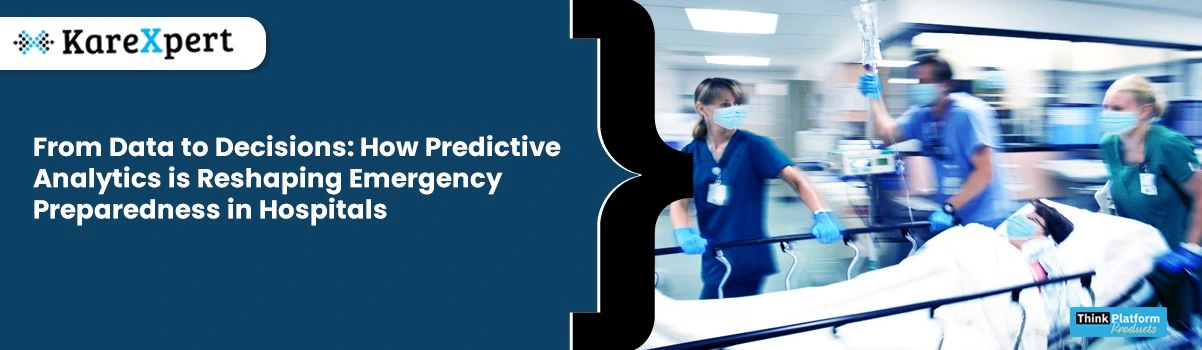
Chapter 1: The Temptation of Predictive Analytics
Predictive analytics is revolutionizing industries from retail to finance, and now healthcare. The promise is seductive: predict patient outcomes, eliminate waste, and reduce cost. Yet healthcare organizations are scrambling to put these tools into practice without considering an essential reality—not all predictive analytics tools are the same, and even fewer are built to address the nuances of healthcare. Choosing the right tools can prevent wasted time, money, compliance risks, and impacts on patient care.
This article explores why the industry needs dedicated solutions, the limitations of generic predictive analytics, and the difficulties they will face in the healthcare sector.
The Allure of “One-Size-Fits-All” Tools
1. Wide Market Interest, Limited Health Care Significance
Predictive analytics platforms designed for various industries tend to feature capabilities such as sales forecasting, market segmentation, and customer behavior tracking. These features might seem appealing but do not easily translate to the workflows of healthcare-centered environments, such as:
– Tracking disease progression.
– Managing treatment regimens.
– Improving hospital patient flow.
2. Longitudinal Data with Limited Understanding of Clinical Context
Generic tools often lack:
– Clinical Relevance: They can’t explain things like comorbidities or complex treatment activity.
– Medical Standards: Many tools do not map with essential healthcare frameworks like ICD-10 or HL7, which are needed for data interoperability.
Why Healthcare Is Different
Predictive analytics in healthcare presents unique problems that require specialized design and application:
1. Complex Data Sets
Healthcare depends on many different sources of data:
– Electronic Health Records (EHRs).
– Diagnostic imaging.
– Wearables and IoT devices.
– Genomic information.
These data types are neither stable nor abundant and are increasingly dependent on one another, making precise integration critical for achieving accurate insights.
2. Regulatory Compliance
Tools need to follow regulations like:
– HIPAA (Health Insurance Portability and Accountability Act): Protecting patient information confidentiality.
– GDPR (General Data Protection Regulation): Ensuring compliance when working with global patient data.
Most generic tools are not compliant with these standards, putting healthcare providers at risk of legal and financial liability.
3. High Stakes
Unlike retail or marketing, where a mistake might mean lost sales, in healthcare:
– Misjudged predictions can produce potentially life-threatening mistakes.
– Maldistribution of resources can create algorithmic overwhelm and delays in transitioning patients out of critical care.
The Dangers of Working Off-the-Shelf Predictive Analytics Packages
1. Inaccurate Predictions
Healthcare data is intrinsically complex, and generic tools may:
– Misinterpret patient symptoms.
– Lack the ability to identify condition-specific associations.
– Provide misleading insights due to limited medical data.
2. Data Silos
Many general analytics platforms do not integrate across healthcare systems and end up contributing to data silos, leading to:
– Lack of a unified patient view.
– Inaccurate predictions.
3. Inability to Scale
As hospitals grow or adopt new technologies, predictive analytics tools must scale seamlessly. Generic solutions often fail to address:
– Increasing patient volumes.
– Growing data streams from high-tech diagnostics and IoT devices.
4. Lack of Real-Time Insights
Healthcare requires the ability to make split-second decisions. Many generic tools are ill-suited for processing data in real-time, delaying vital actions.
What Should a Predictive Analytics Tool Have to Be Ready for Healthcare?
1. Domain-Specific Training
Healthcare tools must be built on models trained with medical data, ensuring predictions align with clinical realities. These models incorporate:
– Disease progression patterns.
– Treatment success rates.
– Patient demographics and history.
2. Interoperability
Tools ready for healthcare deliver integrated workflows with EMRs, diagnostic equipment, and hospital management systems. They adhere to standardized procedures like HL7 and FHIR (Fast Healthcare Interoperability Resources).
3. Regulatory Compliance
These tools are designed to conform to:
– Patient Privacy Laws: Ensuring data confidentiality.
– Data Security Protocols: Protecting sensitive information.
– Healthcare-Specific Reporting Requirements: Meeting industry standards.
4. Explainability
Healthcare professionals must understand the process behind predictions. Healthcare-specific tools provide transparency by:
– Explaining the reasons for predictions.
– Indicating confidence levels in generated insights.
Real-Life Examples: The Good and Bad
1. A Success Story: Predicting Sepsis with Specialized Analytics
A hospital in the United States used a predictive tool customized for early sepsis detection. The tool analyzed patient vitals and lab results to flag high-risk cases within minutes, resulting in:
– A 25% decrease in mortality due to sepsis.
– Optimized staff productivity and resource utilization.
2. Strictly Operational: The Case of Misused Generic Tools
A hospital implemented a standardized analytics platform to forecast patient admission volumes. However:
– The tool failed to account for seasonal disease trends, leading to staffing shortages during flu season.
– Weak EMR integration caused data inaccuracies, creating costly inefficiencies.
The hospital later switched to a healthcare-specific solution, but not before incurring significant financial losses.
What to Look for in Predictive Analytics Tools
When selecting predictive analytics tools, healthcare providers should prioritize the following features:
1. Customizable Algorithms
Algorithms tailored to specialties like:
– Cardiology.
– Oncology.
– Ophthalmology.
2. Real-Time Processing
Essential for applications like:
– Optimizing energy release strategies.
– Identifying patient deterioration in ICUs.
3. User-Friendly Interface
Intuitive dashboards minimize training time and enhance usability for frontline healthcare employees.
4. Scalability
Tools must accommodate new treatment methods, monitoring technologies, and increasing data volumes.
Predictive Analytics in Healthcare: The Future
1. AI and Machine Learning
Advanced AI models will enable:
– Genetic data-based precision medicine.
– Patient-specific chronic disease management.
2. Integration with Wearables
Predictive analytics tools will use real-time data from wearable devices to deliver proactive care.
3. Population Health Management
Advanced analytics will help identify at-risk populations and design public health interventions to prevent disease outbreaks.
Conclusion: Make the Right Choice, Save Lives
Predictive analytics has great potential to transform healthcare—when the right tools are selected. Generic “one-size-fits-all” solutions may be tempting due to their ease of deployment and lower cost, but they pose significant risks, including ineffective care, false diagnoses, and patient harm.
Healthcare providers must invest in role-specific tools purpose-built for the domain—solutions that understand the complexity of medical data, meet regulatory requirements, and deliver meaningful insights in real-time.
In healthcare, the stakes are too high for half measures. With the right predictive analytics tools, hospitals can fully leverage data-driven decision-making, improving outcomes for both patients and providers.